“BREAD Vision” utilizes vision software in conjunction with a BREAD system to judge physical characteristics and analyze the material characteristics of an object, and then return a pass or fail. The device is designed to be an open-source, affordable option for quality control applications.
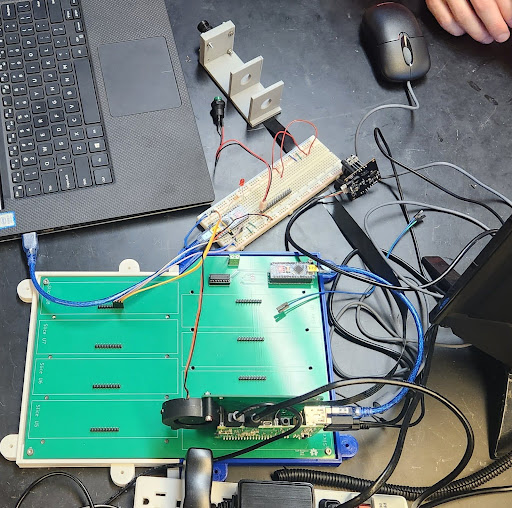
To accomplish this goal, seven functional requirements were created to be completed in four months. The goals are as follows: The entire device will have design files originated entirely from free and open source software. The Raspberry PI will communicate its results with the BREAD loaf and to another BREAD slice. Multiple vision programs can be run from the same camera, i.e. color detection and object detection. The device can identify object defects by detecting inconsistencies in its color, i.e. dents, bruises, discoloration. The OpenCV slice does not connect to the internet. The PI will be able to send meaningful information about spectroscopy of an object. The spectrometer will have a consistent mount. A physical slice will be made. Support for USB cameras will be added. At the end of the semester, all of these goals were reached.
Additionally, three value added goals were created and are as follows: The PI will be able to log meaningful data for future review. The PI will be able to analyze two input streams concurrently. The PI was able to successfully create and store a log file of the printout of each call to a detection module with information on what was detected. Support for both a USB and PI cam was added as well. The only goal that was not reached was updating previous documentation. This will be made a priority for the next semester.
In this system, the Raspberry Pi communicates with the BREAD loaf by establishing an I2C connection and sending its results as responses to commands. The main Python program in the Pi is responsible for handling this communication and can call multiple Python modules to analyze images and send the results back to the BREAD loaf.
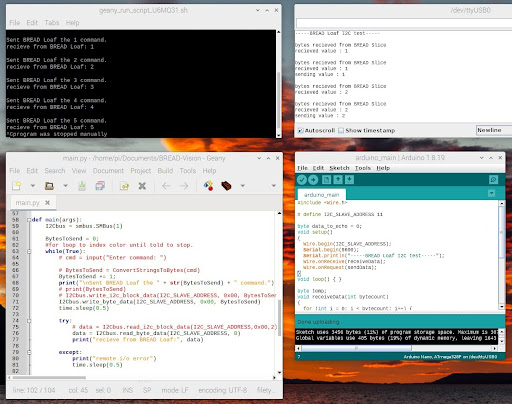
A Pi camera can further expand these capabilities. Color detection can be accomplished through the use of the libraries OpenCV, numpy, time, and the PiCamera library. With a given color that the object is supposed to be, the current integration compares a point central on the camera window to the tolerance computed from the given nominal value and determines if the image from the camera falls within specifications. This can easily be expanded to incorporate multiple different points with different tolerance values for each point.
The object detection system was redone this semester, moving to YOLO v8 as our object recognition software. After training a dataset using Roboflow and uploading the weights to the Pi, our software was able to detect a Starbucks cup. By using a strict training dataset of images of our target in one position, we were able to get the program to detect defects in the target, in our case a Starbucks cup. Missing lids and dents changed the confidence of the system by about 10%, allowing us to set it so that it will not detect the cup when these defects are present.
Utilizing a diffraction spectroscope and an M12 CCTV zoom lens, a spectrometer can be integrated into the Raspberry Pi. This spectrometer, once calibrated, is able to view the specific operating wavelengths of light sources or the absorption bands of a material, allowing one to view the specific characteristics of a material. Many functions and libraries are required to make this spectrometer work, and all the code is available in the OSF repository.
To increase stability and usefulness of the spectrometer with the BREAD system, the information on the spectrometer can be compared to given tolerance values for either intensity, wavelength, or both, ensuring that the final command sent to the LOAF is small enough to be sent over I2C. In addition to this, a mount for the spectrometer has been created to provide additional stability for the system, directly coupling the spectroscope to the lens. This mount can be 3D printed and is accessible in the OSF Repository.
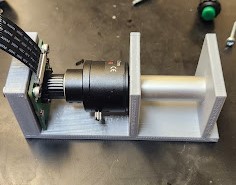
This semester, a physical slice was also added to the system to fully integrate BREAD Vision into the BREAD family. This slice conforms to the requirements of the LOAF, and allows for I2C connections to the rest of the LOAF. It also allows a fan to be mounted on the slice that runs off the 5V pins to improve cooling to the system. All necessary ports are easily accessible and labeled, and the PI can be removed at any time via headers while also ensuring a stable connection for the PI when installed on the slice. This slice does not feature an Arduino as is standard for BREAD, but instead sends data directly to another Arduino on the LOAF.
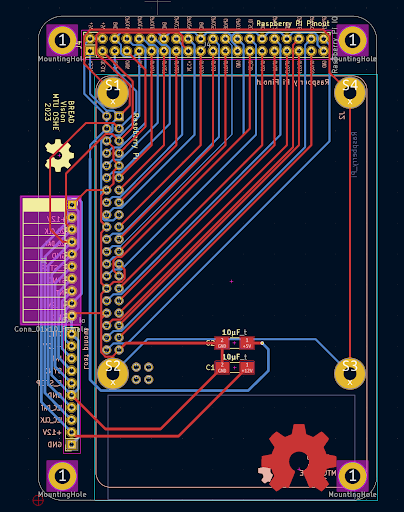
To replicate our system, the first step is to acquire a BREAD system and a Raspberry Pi 4, along with a compatible camera that works with the Pi. Before installing OpenCV, it is essential to install Raspberry Pi Bullseye 3.10+ to gain access to the PiCamera2 library.
Note that if Python is reinstalled, all libraries, including OpenCV, must be reinstalled as well. A complete list of the required libraries can be found in the OSF repository. Once all of the necessary libraries have been installed, simply run main.py.
The color integration and object detection modules are also compatible with a USB camera. Simply plug in the camera into the Pi and run mainUSBcam.py. mainCombined.py can also be used for using both the USB and Pi cameras. When doing either of these modules, it may be better to retrain your Roboflow weights using pictures from the USB camera in order to have a more accurate model when analyzing images from the USB camera.
Depending on the available cameras, the code in the OSF repository can be configured to output spectrograms or compare color values. By following these steps, anyone can replicate our system and utilize it for affordable and accessible quality control applications. The OSF repository can be found here: https://osf.io/j8y9g/
Component | Price |
Raspberry PI 4 | 55$-105$ |
USB Camera (Optional) | 10-25$ |
PI Camera | 30$ |
Diffraction spectroscope (Required for Spectrometer only) | 30$ |
M12 CCTV zoom lens (Required for Spectrometer only) | 15$ |
BREAD Vision Slice + Components | 30$ |